Story
Ā
Object classification is at the heart of modern computer vision applications. From self-driving cars to real-time surveillance systems, the ability to detect, classify, and act upon objects in the visual world has become critical. Among the tools enabling this transformation, YOLOv5 (You Only Look Once, version 5) stands out as an efficient and accessible solution. In this blog, weāll explore YOLOv5ās features, its implementation for object classification, and its real-world applications.
Ā
What is YOLOv5?
YOLOv5 is an advanced object detection algorithm developed by Ultralytics. It follows the legacy of the YOLO family, renowned for its real-time performance, but introduces significant enhancements in usability, speed, and accuracy. Built on PyTorch, YOLOv5 simplifies model training and deployment, making it the go-to choice for researchers and developers worldwide.
Ā
Get PCBs for Your Projects Manufactured
Ā
You must check out PCBWAY for ordering PCBs online for cheap!
Ā
You get 10 good-quality PCBs manufactured and shipped to your doorstep for cheap. You will also get a discount on shipping on your first order. Upload your Gerber files onto PCBWAY to get them manufactured with good quality and quick turnaround time. PCBWay now could provide a complete product solution, from design to enclosure production. Check out their online Gerber viewer function. With reward points, you can get free stuff from their gift shop. Also, check out this useful blog on PCBWay Plugin for KiCad from here. Using this plugin, you can directly order PCBs in just one click after completing your design in KiCad.
Ā
Key Features of YOLOv5:
Ā
Efficiency: YOLOv5 processes images in a single pass through its neural network, ensuring real-time results.Ā Versatility: Available in multiple model sizes (e.g., YOLOv5s, YOLOv5m), it can adapt to various hardware constraints.Ā Accuracy: By integrating innovations like CSPNet and PANet, YOLOv5 achieves exceptional detection and classification accuracy.
Ā
How YOLOv5 Works for Object Classification
YOLOv5 combines object detection and classification in a seamless process. Hereās how it works step-by-step:
Ā
Image Processing: YOLOv5 divides the input image into a grid system. Each grid cell is responsible for detecting objects within its boundaries and assigning them class labels.
Ā
Bounding Box Prediction: For each grid cell, YOLOv5 predicts bounding boxes and confidence scores, ensuring precise localization of objectsĀ
Ā
Class Probability: YOLOv5 assigns class probabilities to each detected object, enabling accurate classification.Ā
Ā
Non-Maximum Suppression (NMS): To eliminate redundant bounding boxes, YOLOv5 applies NMS, retaining only the most confident predictions for each object.Ā
Ā
Output: The final output includes bounding box coordinates, class labels, and confidence scores for detected objects.
Ā
Step-by-Step Guide to Using YOLOv5 for Object Classification
1. Install the Python Environment
If Python is not already installed on your system, download and install it from the official website: https://www.python.org/.
Ā
During installation, ensure the "Add Python to PATH" option is checked to avoid configuration issues.
Ā
Tip: If Python is already installed, you can verify by running the following command in the terminal or command prompt:
Ā
python --version
Ā
2. Install the Required Libraries
Open the Command Prompt by pressing Win+R, typing "cmd", and hitting Enter.
Ā
Execute the following commands one by one to install the necessary libraries:
Ā
pip install numpy
pip install yolov5
Ā
Ensure that your pip installation is up-to-date by running:
Ā
pip install --upgrade pip
Ā
3. Load the script
Open the given script in VS Code.
import cv2
import torch
import time
from yolov5 import YOLOv5
# Initialize webcam
cap = cv2.VideoCapture(0) # 0 = default webcam
# Load the YOLOv5 model
model_path = "yolov5s.pt" # Path to your YOLOv5 model (make sure it's downloaded)
device = torch.device("cuda" if torch.cuda.is_available() else "cpu")
model = YOLOv5(model_path, device=device)
while True:
ret, frame = cap.read() # Capture frame from webcam
if not ret:
print("Failed to grab frame")
break
# Record processing start time
start_time = time.time()
# Perform inference (no gradients needed for inference -> faster)
with torch.no_grad():
results = model.predict(frame, size=640) # Resize input to 640x640
# Get annotated results
annotated_frame = results.render()
# Make a writable copy to draw on
frame_to_show = annotated_frame[0].copy()
# Record processing end time
end_time = time.time()
# Calculate FPS
processing_time = end_time - start_time
fps = 1 / processing_time if processing_time > 0 else 0
# Draw FPS on the image
cv2.putText(frame_to_show, f"FPS: {fps:.2f}", (10, 30),
cv2.FONT_HERSHEY_SIMPLEX, 1, (0, 255, 0), 2, cv2.LINE_AA)
# Display the frame
cv2.imshow("YOLOv5 Detection", frame_to_show)
# Print details to terminal
print(f"Processed frame in {processing_time:.4f} seconds, FPS: {fps:.2f}")
# Exit when 'q' key is pressed
if cv2.waitKey(1) & 0xFF == ord('q'):
break
# Release resources
cap.release()
cv2.destroyAllWindows()
4. Execute
Ā
Once the script is loaded, press F5 to run it.
Ā
Observe the recognition screen, where the YOLOv5 model processes the camera feed and displays classification results in real time.
Ā
Ā
Applications of YOLOv5 in Object Classification
Ā
YOLOv5ās versatility has unlocked applications across various industries:
Ā
Autonomous Vehicles: Detect and classify objects like pedestrians, vehicles, and traffic signs for safe navigation.Ā
Ā
Healthcare: Identify anomalies in medical images, such as tumors in X-rays and MRIs.Ā
Ā
Retail: Automate inventory management by detecting and classifying products on shelves.Ā
Ā
Agriculture: Monitor crops and identify pests using drone imagery.Ā
Ā
Surveillance: Real-time detection of suspicious activities and unauthorized access.Ā
Ā
Wildlife Conservation: Track and identify endangered species for ecological studies.
Ā
Why Choose YOLOv5?
YOLOv5 offers several advantages over other object detection algorithms like Faster R-CNN and SSD:
Ā
Speed: Processes images in milliseconds, making it ideal for time-critical applications.Ā
Ā
Compactness: Requires fewer computational resources, suitable for edge devices.Ā
Ā
Ease of Use: Streamlined training and deployment process, even for beginners.
Ā
Challenges and Future Prospects
Ā
Challenges:
Ā
Detection of very small objects can be less accurate.Ā Performance depends heavily on the quality of training data.
Ā
Future Prospects:
Ā
Integration with multimodal learning for richer context understanding.Ā Development of semi-supervised techniques to reduce reliance on labeled datasets.Ā Enhanced support for real-time video analytics and edge computing.
Ā
Conclusion
YOLOv5 has redefined the boundaries of object classification with its speed, accuracy, and simplicity. From life-saving applications in healthcare to transformative technologies in autonomous driving, YOLOv5 continues to shape the future of computer vision. Whether youāre a researcher or a developer, diving into YOLOv5 is an exciting journey that can open doors to endless possibilities.
Ā
So, get started todayālet YOLOv5 empower your next innovation!
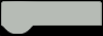