
IoT AI-driven Food Irradiation Dose Detector w/ Edge Impulse
Even though food irradiation improves food hygiene, spoilage reduction, and extension of shelf-life, it should be regulated strictly to avoid any health risks and nutritional value drops. However, small businesses in the food industry lack a budget-friendly and simple way to detect food irradiation doses after treating food with ionizing energy, especially for animal (livestock) feed. Therefore, I decided to build an AI-driven IoT device predicting food irradiation doses based on weight, color (visible light), and emitted ionizing radiation.
Ionizing radiation is a nonthermal process utilized to achieve the preservation of food. At a maximum commercial irradiation dose of 10 kGy, irradiation does not impart heat to the food, and the nutritional quality of the food is generally unaffected. The irradiation process can reduce the microbial contamination of food, resulting in improved microbial safety as well as the extended shelf-life of the food[1]. Irradiation also benefits the consumer by reducing the risk of severe health issues caused by foodborne illnesses. Food irradiation has three categories: low-dose (radurization), medium-dose (radicidation), and high-dose (radappertization). Low dose irradiation (under 1 kGy) inhibits the sprouting of produce (onion, potato, and garlic); retards the ripening and fungi deterioration of fruits and vegetables (strawberry, tomato, etc.), and promotes insect disinfestations in cereals and vegetables. Medium dose irradiation (between 1 and 10 kGy) controls the presence of pathogenic organisms, especially in fruit juices; retards the deterioration of fish and fresh meat; and reduces Salmonella in poultry products, similar to pasteurization. High dose irradiation (over 10 kGy) is rather significant to the sterilization of health and personal hygiene products[2].
Since foods treated with ionizing radiation should be adequately labeled under the general labeling requirements, consumers can make their own free choice between irradiated and non-irradiated food. However, unfortunately, some countries do not apply strict regulations for irradiated foods, especially for animal feed. Therefore, detecting proper irradiation doses can be arduous for small businesses in the food industry due to governments not incentivizing strictly regulated food irradiation processes. Since irradiation can engender certain alterations that can modify the chemical composition and nutritive values of food, depending on the factors such as irradiation dose, food composition, packaging, and processing conditions such as temperature and atmospheric oxygen saturation[2], unsupervised food irradiation portends health issues.
After scrutinizing recent research papers on food irradiation, I decided to utilize ionizing radiation, weight, and visible light (color) measurements denoting the applied irradiation dose so as to create a budget-friendly and accessible device to predict food irradiation dose levels in the hope of assisting small businesses in checking compliance with existing regulations on food irradiation.
Although ionizing radiation, weight, and visible light (color) measurements provide insight into detecting food irradiation doses, it is not possible to conclude and interpret food irradiation doses precisely by merely employing limited data without applying complex algorithms since food irradiation dose levels fluctuate depending on processing techniques, food characteristics, and equipment. Therefore, I decided to build and train an artificial neural network model by utilizing the theoretically assigned food irradiation dose classes to predict food irradiation dose levels based on ionizing radiation, weight, and visible light (color) measurements. Since I could not apply ionizing radiation directly to foods by emitting Gamma rays, X-rays, or electron beams, I exposed foods to sun rays as a natural source of radiation for estimated periods.
Since Beetle ESP32-C3 is an ultra-small size development board intended for IoT applications, that can easily collect data and run my neural network model after being trained to predict food irradiation doses, I decided to employ Beetle ESP32-C3 in this project. To obtain the required measurements to train my model, I utilized a Geiger counter module (Gravity), an I2C weight sensor (Gravity), and an AS7341 11-channel visible light sensor (Gravity). Since Beetle ESP32-C3 is equipped with an expansion board providing the GDI display interface, I connected an SSD1309 OLED transparent screen (Fermion) to display the collected data.
After collecting data successfully, I developed a PHP web application that obtains the transmitted data from Beetle ESP32-C3 via HTTP GET requests, logs the received measurements in a given MySQL database table, and lets the user create appropriately formatted samples for Edge Impulse.
After completing my data set and creating samples, I built my artificial neural network model (ANN) with Edge Impulse to make predictions on food irradiation dose levels (classes) based on ionizing radiation, weight, and visible light (color) measurements. Since Edge Impulse is nearly compatible with all microcontrollers and development boards, I had not encountered any issues while uploading and running my model on Beetle ESP32-C3. As labels, I employed the theoretically assigned food irradiation dose classes for each data record while collecting and logging data:
Regulated
Unsafe
Hazardous
After training and testing my neural network model, I deployed and uploaded the model on Beetle ESP32-C3. Therefore, the device is capable of detecting precise food irradiation dose levels (classes) by running the model independently without any additional procedures.
Lastly, to make the device as robust and compact as possible while experimenting with a motley collection of foods, I designed a Hulk-inspired structure with a moveable visible light sensor handle (3D printable).
So, this is my project in a nutshell 😃
Click here to inspect code files, STL files, and instructions.







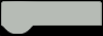